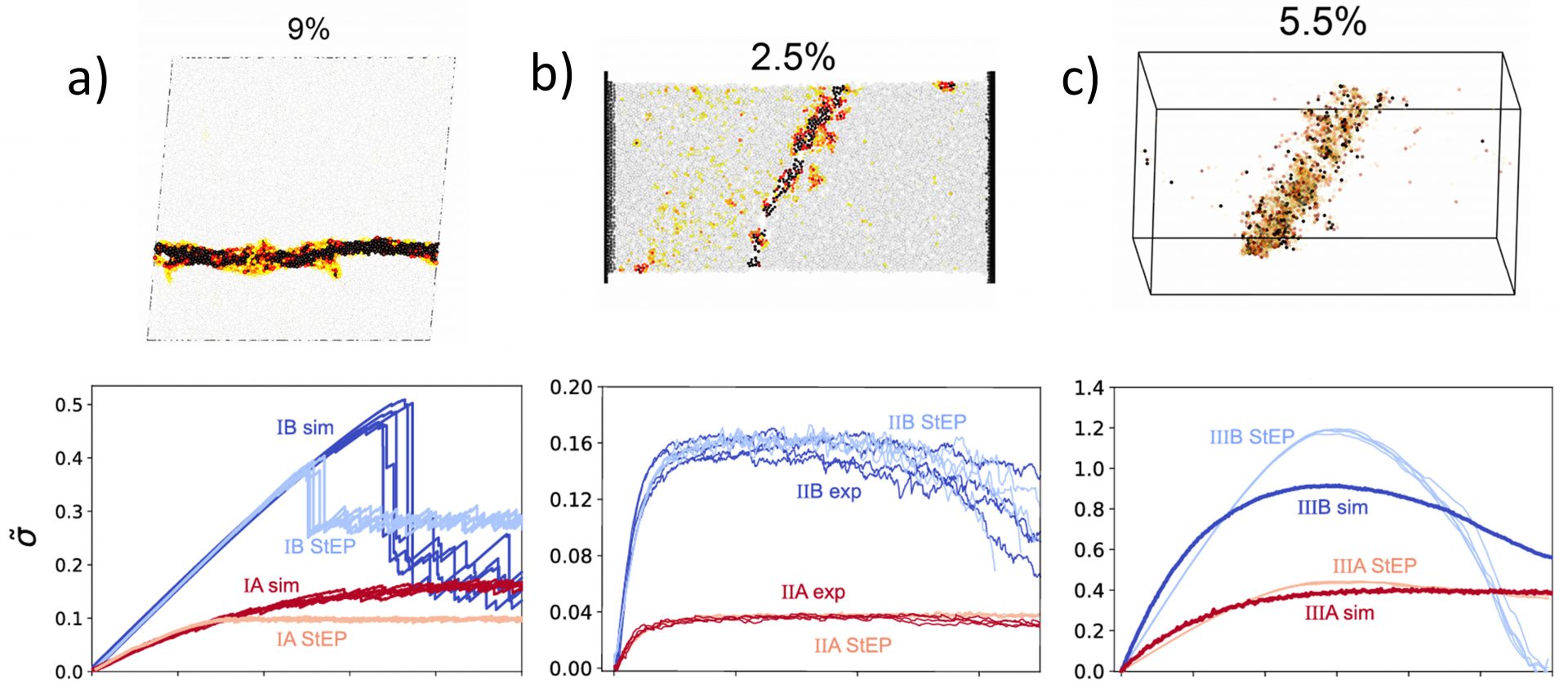
Shear bands formation in different disordered solids are well-captured by our mesoscopic StEP model. From left to right: simulated atomic glasses (left), experimental granular packing (middle), and a simulated polymer glass (right). The particles are colored according to their local strain with darker red indicating higher strain, and the applied strain localizes in all three cases. The bottom row has the stress/strain response of the three systems measured in simulations or experiments compared to the StEP model for the same three systems, showing very good agreement.
Disordered particulate solids are ubiquitous in items ranging from plastic to concrete. Despite their prevalence, applications can be limited because they are often brittle. In contrast, ductile materials can be deformed smoothly and significantly without fracturing. Strategies for tuning ductility of disordered solids are empirical and system-specific.
Liu, Riggleman and Durian used computer simulations of atomic and polymeric glasses and laboratory experiments on granular packings to develop a general Structuro-Elasto-Plastic (StEP) framework for understanding large-scale deformation of disordered solids in terms of the system-specific interplay between local structure, local rearrangements and larger-scale elasticity.
The researchers first used known empirical strategies to tune each system to tune each system to exhibit different degrees of strain localization, which is the tendency for deformation to concentrate in specific areas rather than being evenly distributed throughout the material. Then they used a machine learning-based descriptor (‘softness’) as a key new quantity. All inputs to their model were independently measured and used to predict the stress-strain curves and the degrees of strain localization without adjustable fitting parameters.
This work could lead to quantitative approaches to designing disordered materials that are more resistant to fracture, a significant advance in materials science.
Xiao, Hongyi, et al. “Identifying microscopic factors that influence ductility in disordered solids.” Proceedings of the National Academy of Sciences 120.42 (2023): e2307552120. https://doi.org/10.1073/pnas.2307552120