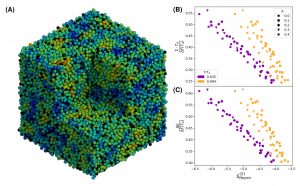
(A) Spatial distribution of particles with different machine-learned softness, strongly correlated with localized particle rearrangements within the glass. (B,C) Rearrangement barriers (entropic and energetic), rescaled to be dimensionless, against the ML-informed excess entropy of thermal configurations. Approximate collapse is observed in the data at the same temperatures relative to the onset of glassy dynamics. Agreement improves as one moves deeper into the supercooled regime.
The properties of glasses – disordered, amorphous materials – can be hard to predict because of this lack of long-range order and the associate properties of crystal symmetry.
Work in this IRG has developed two fundamental descriptors to describe glass properties. The first of these – softness – is a machine-learning derived descriptor that characterizes structural defects in glasses and predicts rearrangements or yield that will occur in disordered materials in response to applied loads. The second – excess entropy – is a thermodynamic quantity that is a simple function of that describes the deviation of atomic arrangements from what would be predicated from ideal gas theory.
This study explored the relationship between these two parameters, in an effort to understand how fundamental thermodynamics via excess entropy can provide insight into a machine-learned, predictive descriptor, softness.
Numerical simulations showed that excess entropy can describe many of the same features as softness, providing a new framework to describe supercooled liquids such as glasses.
Graham, I. R., Arratia, P. E. and Riggleman, R. A., Exploring the relationship between softness and excess entropy in glass-forming systems. Journal of Chemical Physics 158 (20), (2023) 10.1063/5.0143603 https://doi.org/10.1063/5.0143603