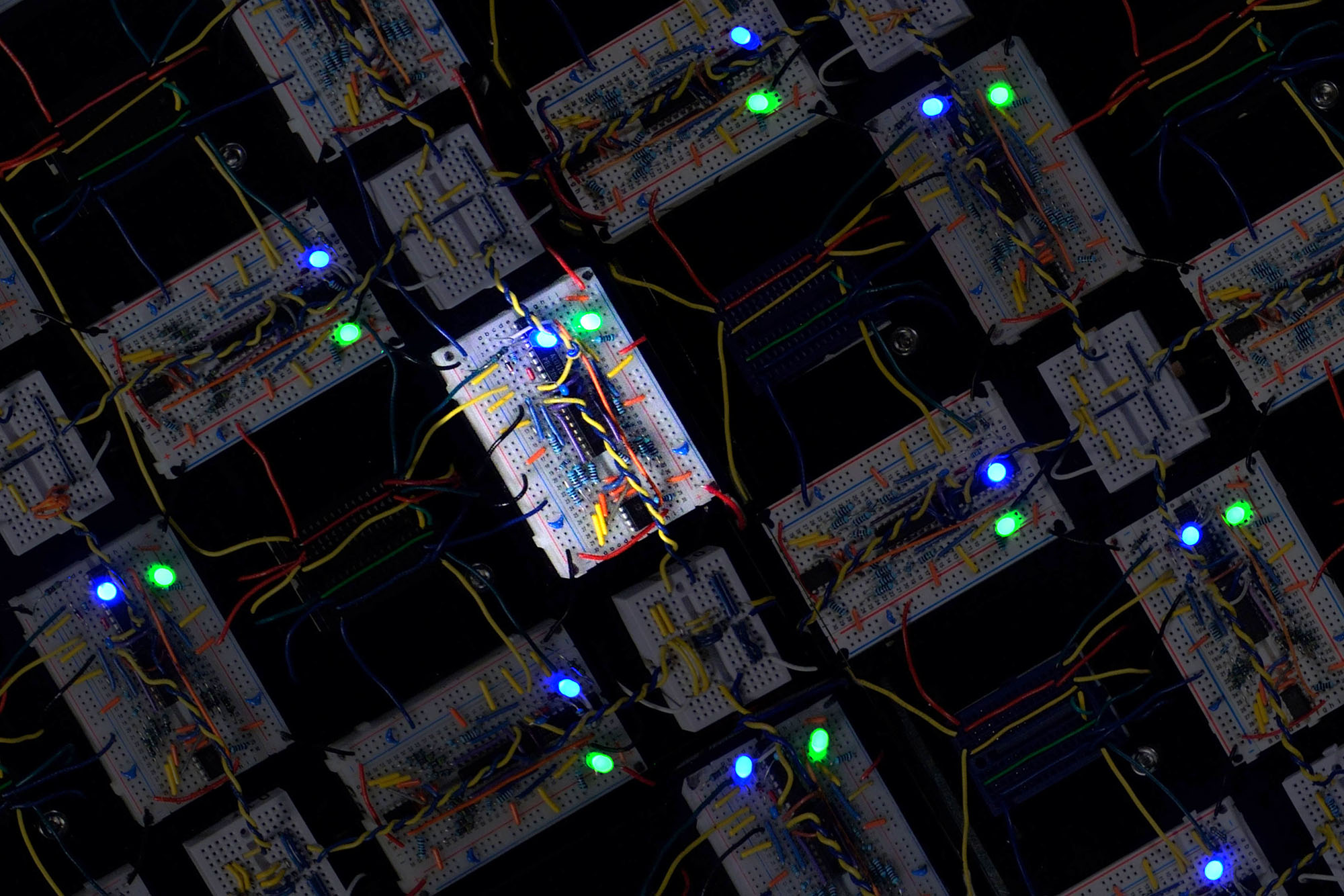
The capabilities of digital artificial neural networks grow rapidly with their size. Unfortunately, so do the time and energy required to train them. By contrast, brains function rapidly and power-efficiently at scale because their analog constituent parts (neurons) update their connections without knowing what all the other neurons are doing; in other words, they update using local rules. Recently introduced analog electronic contrastive local learning networks (CLLNs) share this important property. However, unlike brains and artificial neural networks, their capabilities were limited and could not grow with size because they are linear. Here, we experimentally demonstrate that nonlinearity enhances machine-learning capabilities in an analog CLLN, establishing a paradigm for scalable learning systems.
read article